By: Jon Williams
Border security management is a critical aspect of national security, encompassing a range of measures aimed at protecting a country’s borders from unauthorized entry and illicit activities. In an increasingly interconnected world, where threats can come from various sources, including terrorism, smuggling, and illegal immigration, the need for effective border security has never been greater.
One promising approach to enhancing border security is through the use of predictive analytics. This technology harnesses the power of data analysis to identify patterns and trends that can help predict future events. By leveraging data from a variety of sources, including surveillance systems, sensors, and intelligence databases, predictive analytics can provide border security agencies with valuable insights into potential threats, allowing them to take proactive measures to mitigate risks.
International border security company, Securiport, explores the concept of predictive analytics in the context of border security management. Lending their insight in advanced security measures, leaders of Securiport discuss how this approach can help enhance border security, improve decision-making processes, and ultimately contribute to a safer and more secure border environment.
Understanding Predictive Analytics
Predictive analytics is a sophisticated data analysis technique that leverages historical data, statistical algorithms, and machine learning to forecast future events. It is a valuable tool used across various industries, including border security management, to anticipate and mitigate potential risks.
“At its core, predictive analytics aims to identify patterns and trends in data that can be used to predict future outcomes,” says an executive from Securiport. “This is achieved through the use of predictive models, which are algorithms trained on historical data to make informed predictions about future events.”
By analyzing past trends and behaviors, predictive analytics can help border security agencies anticipate potential threats and take proactive measures to prevent them. In border security management, predictive analytics can play a crucial role in enhancing decision-making processes.
By providing insights into potential threats, predictive analytics enables agencies to allocate resources more effectively, prioritize security measures, and respond swiftly to emerging risks. Overall, predictive analytics is a powerful tool that can significantly enhance border security efforts by enabling agencies to anticipate and address potential threats before they occur.
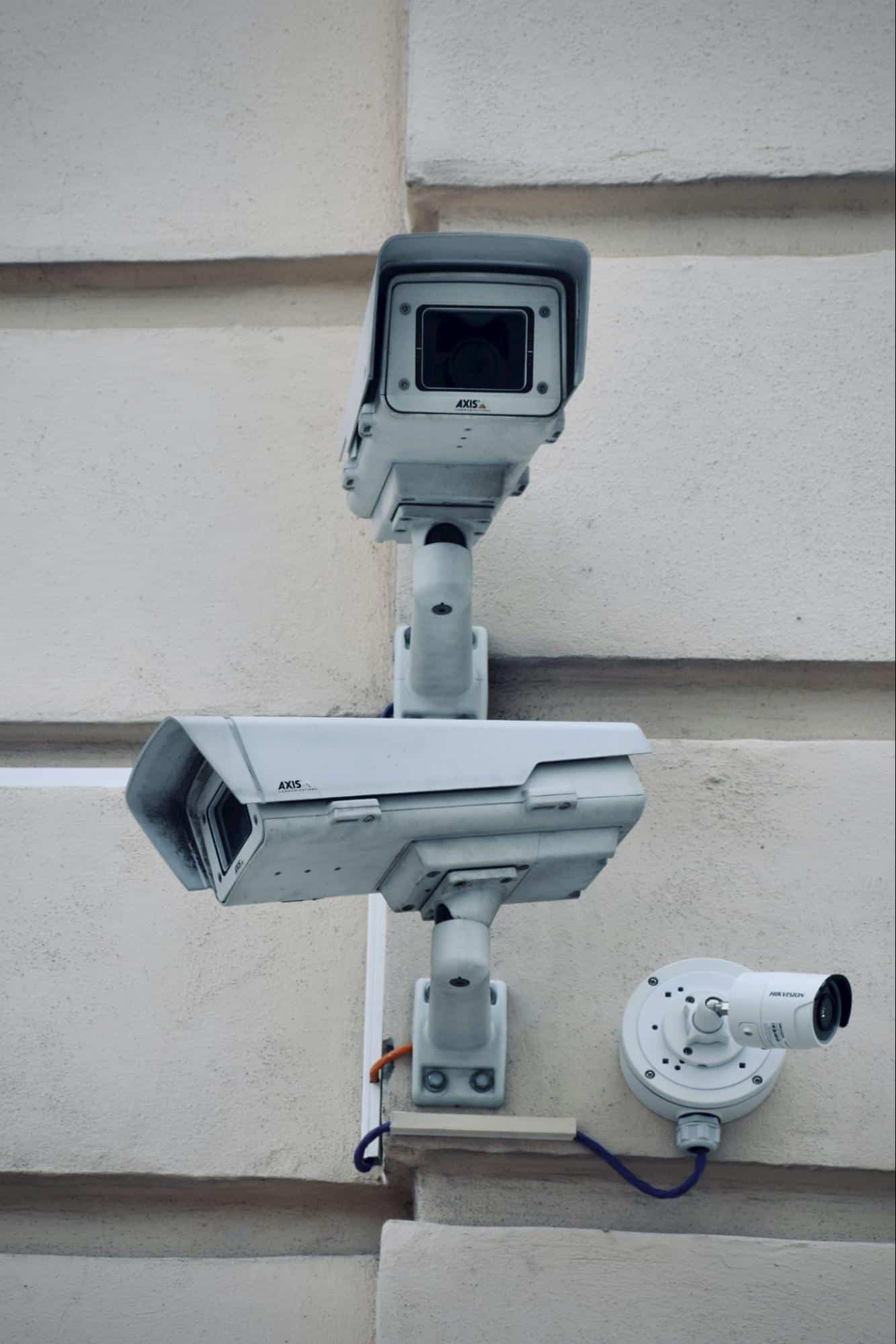
Applications of Predictive Analytics in Border Security
Border security management faces numerous challenges, including illegal immigration, smuggling, and terrorism.
Notes a Securiport Tech Manager, “These threats are dynamic and often difficult to anticipate, requiring border security agencies to constantly adapt their strategies to protect national borders effectively.”
Predictive analytics offers a powerful solution to these challenges by enabling border security agencies to anticipate and mitigate potential threats. By analyzing data from a variety of sources, including surveillance systems, intelligence databases, and social media, predictive analytics can identify patterns and trends that may indicate potential security risks. This enables agencies to allocate resources more effectively, target high-risk areas, and deploy personnel where they are most needed.
Several tools and technologies are used in border security operations to implement predictive analytics. These include data mining techniques, machine learning algorithms, and predictive modeling software. These tools can analyze vast amounts of data in real time, providing border security agencies with timely and actionable insights to enhance their decision-making processes. Overall, predictive analytics has the potential to revolutionize border security management by enabling agencies to stay ahead of emerging threats and protect national borders more effectively.
Data Sources and Integration
Border security management relies on a variety of data sources to gather information and intelligence. These sources include sensor data from surveillance systems, satellite imagery, social media feeds, and government databases. Each of these sources provides valuable insights that can help border security agencies anticipate and respond to potential threats.
Integrating these diverse data sources poses several challenges. One of the main challenges is the heterogeneity of the data, as each source may use different formats and structures. Ensuring data quality and consistency is also crucial, as inaccurate or incomplete data can lead to erroneous predictions and decisions.
“Data governance plays a critical role in addressing these challenges. Establishing clear policies and procedures for data collection, storage, and sharing can help ensure that data is accurate, reliable, and consistent across different sources,” says a Securiport leader.
Privacy considerations are paramount, especially when dealing with sensitive border security data. Implementing robust security measures and adhering to legal and regulatory requirements can help protect sensitive information and maintain public trust in border security efforts.
Building Effective Predictive Models
Building predictive models for border security involves several key steps. The first step is to define the predictive task, which includes identifying the specific threat or risk to be predicted. Next, data collection and preprocessing are carried out to prepare the data for analysis. This may involve cleaning the data, handling missing values, and encoding categorical variables.
Once the data is ready, the next step is to select the appropriate algorithms for building the predictive model. The choice of algorithm depends on the nature of the data and the predictive task. Common algorithms used in predictive analytics include decision trees, random forests, and neural networks.
After selecting the algorithm, the model is trained on the historical data to learn patterns and relationships. Model evaluation and validation are then carried out to assess the performance of the model. This involves splitting the data into training and testing sets to evaluate the model’s accuracy and generalization to new data.
The model is deployed in real-world border security scenarios. Continuous monitoring and iteration are essential to ensure that the model remains effective and accurate over time. Regular updates and improvements to the model are made based on new data and insights gained from its deployment.
The future of border security management lies in harnessing the power of data-driven predictive analytics. By leveraging historical data and advanced algorithms, border security agencies can anticipate and mitigate potential threats more effectively. Collaboration between data scientists, security agencies, and policymakers is crucial to ensuring the successful implementation of predictive analytics in border security.
Published By: Aize Perez